The influence of blockchain technology on circular economy implementation in the automotive sector: From a GMM model to a new machine learning algorithm
ORCID
- Wai Mun Lim: 0000-0002-3115-9175
Abstract
This investigation explores the integration of blockchain technology (BCT) with circular economy (CE) principles within the automotive sector, leveraging a dataset from the years 2011 to 2019. Employing advanced analytical techniques, including machine learning models and the system generalized method of moments (GMM), the study meticulously assesses BCT's impact on CE practices over the specified period. The dataset, curated from esteemed sources such as CSRHub, Thomson Reuters, and Bloomberg, enhances the reliability and validity of our analysis. Results indicate a positive influence of BCT on the adoption and effectiveness of CE practices in the automotive industry, suggesting that CE practices can bolster firm performance. Notably, the analysis reveals that support vector machines (SVM) and neural networks (NNs) exhibit superior efficacy over the random forest (RF) model in capturing the nuances of the BCT-CE interplay. This is evidenced by their lower root-mean-square error (RMSE) and mean absolute error (MAE), signifying greater predictive accuracy. The findings illuminate BCT's potential to revolutionize CE practices, optimize resource use, and foster sustainability in the automotive field.
DOI
Publication Date
2024-11-05
Publication Title
Business Strategy and the Environment
ISSN
0964-4733
Embargo Period
2026-11-05
Keywords
automotive industry, blockchain technology, circular economy, machine learning, system GMM
Recommended Citation
Lin, W., Leow, N., Lim, W., Ho, M., Yong, J., & Pek, C. (2024) 'The influence of blockchain technology on circular economy implementation in the automotive sector: From a GMM model to a new machine learning algorithm', Business Strategy and the Environment, . Available at: 10.1002/bse.4003
This item is under embargo until 05 November 2026
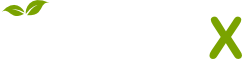
- Citations
- Citation Indexes: 1
- Usage
- Abstract Views: 14
- Captures
- Readers: 19
- Mentions
- News Mentions: 1