Abstract
The development of intelligent music production tools has been of growing interest in recent years. Deep learning approaches have been shown as being a highly effective method for approximating individual audio effects. In this work, we propose an end-to-end deep neural network based on the Wave-U-Net to perform automatic mixing of drums. We follow an end-to-end approach where raw audio from the individual drum recordings is the input of the system and the waveform of the stereo mix is the output. We compare the system to existing machine learning approaches to intelligent drum mixing. Through a subjective listening test we explore the performance of these systems when processing various types of drum mixes. We report that the mixes generated by our model are virtually indistinguishable from professional human mixes while also outperforming previous intelligent mixing approaches.
Publication Date
2021-03-01
Publication Title
Journal of the Audio Engineering Society
Volume
69
Issue
3
Publisher
Audio Engineering Society
ISSN
0004-7554
Embargo Period
2024-11-22
First Page
142
Last Page
151
Recommended Citation
Martinez Ramirez, M., Stoller, D., & Moffat, D. (2021) 'A Deep Learning Approach to Intelligent Drum Mixing with the Wave-U-Net', Journal of the Audio Engineering Society, 69(3), pp. 142-151. Audio Engineering Society: Available at: 10.17743/jaes.2020.0031
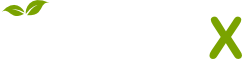
- Citations
- Citation Indexes: 17
- Usage
- Downloads: 19
- Abstract Views: 17
- Captures
- Readers: 23
- Mentions
- News Mentions: 1