ORCID
- Md Faysal: 0000-0002-6674-6249
Abstract
Narcissism is a multifaceted construct often linked to pathological conditions whose neural correlates are still poorly understood. Previous studies have reported inconsistent findings related to the neural underpinnings of narcissism, probably due to methodological limitations such as the low number of participants or the use of mass univariate methods. The present study aimed to overcome the previous methodological limitations and to build a predictive model of narcissistic traits based on neural and psychological features. In this respect, two machine learning-based methods (Kernel Ridge Regression and Support Vector Regression) were used to predict narcissistic traits from brain structural organization and from other relevant normal and abnormal personality features. Results showed that a circuit including the lateral and middle frontal gyri, the angular gyrus, Rolandic operculum, and Heschl's gyrus successfully predicted narcissistic personality traits ( p < 0.003). Moreover, narcissistic traits were predicted by normal (openness, agreeableness, conscientiousness) and abnormal (borderline, antisocial, insecure, addicted, negativistic, machiavellianism) personality traits. This study is the first to predict narcissistic personality traits via a supervised machine learning approach. As such, these results may expand the possibility of deriving personality traits from neural and psychological features.
Publication Date
2023-07-31
Publication Title
Social Neuroscience
Volume
18
Issue
5
ISSN
1747-0919
Keywords
Kernel Ridge Regression, Narcissism, Narcissistic personality disorder, Neuroimaging, Support vector regression, MRI, sMRI, Personality, Machine learning
First Page
257
Last Page
270
Recommended Citation
Jornkokgoud, K., Baggio, T., Faysal, M., Bakiaj, R., Wongupparaj, P., Job, R., & Grecucci, A. (2023) 'Predicting narcissistic personality traits from brain and psychological features: A supervised machine learning approach', Social Neuroscience, 18(5), pp. 257-270. Available at: 10.1080/17470919.2023.2242094
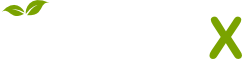
- Citations
- Citation Indexes: 9
- Usage
- Downloads: 57
- Abstract Views: 10
- Captures
- Readers: 25
- Mentions
- Blog Mentions: 1
- News Mentions: 1